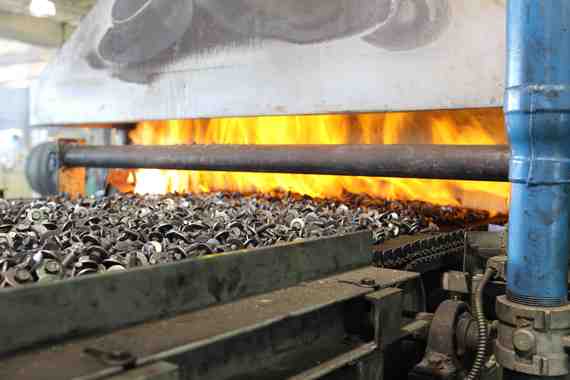
By Jon Sobel
Let's tone down the hype about the Industrial Internet of Things. While the concept shows promise--building smart machines that use sensors and Internet connectivity to improve performance and catch problems--the far more pressing opportunity is learning to make better use of the mountains of data that factories already generate each year, data that manufacturers today often discard after a production run or store unexamined.
I recently visited an auto plant that is facing enormous pressure to reduce defects in a critical component. For each part produced, sensors at a single machine measure 50,000 data points looking for defects, while other machines capture x-ray and heat treatment data. Separate databases track supplier data and quality data.
Despite all that data, too many defective parts escape detection and get installed into vehicles. The plant manager wants to identify correlations within these heterogeneous sets of data, but lacks the tools to collect, condition, and analyze the information.
Last summer he hired interns from a nearby university to look through the data with Excel spreadsheets in search of useful insights. His conclusion: "We have two orders of magnitude more data than we had five years ago," he told me. "We need three orders of magnitude improvement in our ability to understand it."
Across industries, companies are grappling with how to make use of production data. They've come to believe there's great value hidden in their data but they haven't yet figured out how to find it. Unlike in industries such as marketing and finance, which have over the past decade developed sophisticated big data tools for turning bits of data into value, few such applications have been built for manufacturing.
In the words of one engineer we know, manufacturing is "DRIP"--data rich, information poor. Manufacturers generate data across massive, distributed operations, but the data lives in silos. Until we can make use of the data we already have, collecting ever more data just buries us deeper.
While many types of factory equipment produce real-time production data, the data is short-lived and little-used. In a typical example, images of a weld are captured by a factory floor camera and scanned to look for defects. Once the part is cleared, or the production run is complete, the data are deleted to make room for the next batch.
Why is this? Factory floor automation has always been dominated by hardware makers, who have adopted few of the innovations commonplace in software-dominated businesses--ubiquitous connectivity, unlimited and inexpensive data storage, and cloud-based processing. The model behind much of the information technology on the factory floor was developed three decades ago when storage was too expensive and data transmission too costly to even think about capturing, storing, and analyzing high-volume data.
Manufacturing is also inherently a higher stakes endeavor than industries like advertising that have embraced big data techniques. Unlike bad advertising, bad manufacturing can kill people. Large-scale manufacturing plants can't run on beta versions of software. A halt on an auto production line can cost thousands of dollars a minute. Defects cost auto manufacturers millions and sometimes billions of dollars.
The opportunity for digitization in manufacturing is massive--it is an $11 trillion sector with very low penetration of digital technologies. Manufacturing dwarfs industries already permeated by digital technology, including telecom ($2 trillion) and advertising ($500 billion). It is larger even than healthcare ($7 trillion), which until recently was nearly as "undigitized" as manufacturing is today.
Move beyond the factory to the industry level and the need for better manufacturing data analytics becomes more compelling. Manufacturers across industries are grappling with faster product cycles, increasingly complex global supply chains, rapidly rising offshore labor costs, mass customization, and regulatory demands for traceability. In industries such as pharmaceutical and food and beverage, new regulations require companies to track and analyze products from the point of manufacture to the point of sale.
Even in the most traditional industries, manufacturers are scrambling to make better use of their data to improve quality and efficiency. Despite investing billions of dollars in quality control, automakers remain beset with quality problems. General Motors has recalled 25 million vehicles so far this year, and Toyota 29 million in the last four years. Even in good years, U.S. automakers regularly set aside reserves equaling half their profit to cover defects.
Google and Yahoo gained huge advantages over incumbent advertising platforms by applying big data techniques that turn consumer mouse clicks into high-value information. In manufacturing, where minor differences in efficiency and productivity can determine which companies thrive and which fail, the first companies that figure out how to convert their data into actionable information will gain a similar advantage.
Jon Sobel is CEO and co-founder of Sight Machine Inc., which provides cloud-based data analytics for manufacturing quality, traceability, and operations.
Original article published at Techonomy.com.